We’ve written a few times about using LLMs to write/enhance prompts (Using LLMs to Optimize Your Prompts, How to Optimize Long Prompts, RecPrompt). Most of these methods use actions like adding, removing, or replacing parts of a prompt, trying the new versions, and iterating to find the best one.
Generally, these methods use a “frozen” (static) unmodified LLM to evaluate the effectiveness of the new prompts. But what if you trained the evaluator LLM? Enter PRewrite! PRewrite comes from a recent paper from Google: PRewrite: Prompt Rewriting with Reinforcement Learning.
PRewrite’s automated prompt engineering framework differentiates itself through using reinforcement learning to refine the LLM used to rewrite prompts.
How so? Let's dive in and see if this new framework will replace prompt engineering.
What is PRewrite
PRewrite is an automated framework for optimizing prompts.
The biggest difference between PRewrite and other automated prompt optimization frameworks is the use of a reinforcement learning loop. This loop enables the Prompt Rewriter to continually improve using a reward computed on the generated output against the ground-truth output.
Simply put, the Prompt Rewriter gets fine-tuned based on previously enhanced prompts.

PRewrite components
As depicted above PRewrite has a few components:
Prompts
Here are a few examples of the prompts used in the PRewrite process.
Initial Prompt: The original hand-written prompt. This is the starting point.
Meta Prompt: Rewrites the initial prompt
Rewritten Prompt: The final rewritten prompt generated by the Prompt Rewriter
Reinforcement learning components
The reinforcement loop consists of two major components, a policy function and rewards.
Policy function
The policy function is an algorithm that guides the Prompt Rewriter model to make decisions that will enhance the prompt, based on a certain reward. It is a probability distribution over a set of potential actions given the current state.
For example, let’s say you want to cross the street at a busy intersection. This is how your internal policy function would run:
- Evaluate the current state (traffic lights, cars, distance) and potential actions (wait, start walking, speed up, slow down) to maximize safety (the reward in this case).
- Through experience you’ll learn which actions lead to maximizing safety (the reward) in different traffic conditions. Continually optimizing your policy function over time.
Back to PRewrite.
The actions to consider are which tokens to add, delete, or modify, based on the current state, which is the Initial Prompt. The policy function is there to guide the prompt rewriter model in making decisions that are expected to maximize the reward, which is determined by the effectiveness of the rewritten prompt in generating accurate outputs.
Rewards
Rewards are used to inform the policy function and Prompt Rewriter about the effectiveness of the newly rewritten prompt, based on the changes made. The researchers explored a few different reward functions:
- Exact Match (EM): Checks if the output exactly matches the ground-truth output
- F1: Combines precision (correct predictions divided by the total number of predictions made) and recall (correct predictions divided by the total number of actual positives) into one metric. For example, with 80% precision (80 correct out of 100 predictions) and approximately 78% recall (70 correct out of 90 positives), the F1 score averages these to evaluate model performance.
- Perplexity: Measures the model's prediction certainty. Lower values indicating the model is less surprised by the sequence of tokens. Low perplexity: “The dog ate a bone”. High perplexity “The dog ate rigatoni ragù”. Lower perplexity is rewarded.
- Perplexity + F1: Combines perplexity (the unexpectedness of the outputwith F1 (evaluating accuracy and completeness), rewarding outputs that are predictable and precise.
- Length difference: Rewards based on the length difference between the output and ground-truth output.
PRewrite flow
Bringing it all together, here is what the flow looks like:
- Start with an initial prompt, p0
- p0 is rewritten by the Prompt Rewriter, using the meta-prompt, to get a set of prompt variants
- All of the variants are fed into an LLM to generate outputs
- The Prompt Rewriter continually gets trained using reinforcement learning based on rewards determined by the effectiveness of the generated output against the ground-truth.
Experiments set up
Datasets: Natural Questions (NQ), SST- 2, and AG’s News
Models used: PaLM 2-S for the Prompt Rewriter and the model that runs the rewritten prompt
Experiment results
Let’s dive right in.

Takeaways
- PRewrite outperforms the original prompt for NQ and AG, but not for SST-2. This is most likely due to the fact that the tasks in SST-2 are extremely simple and don’t have a lot of room for improvement over the initial prompt
- As a point of reference, the SST-2 dataset focuses on sentiment analysis derived from movie reviews, e.g., “contains no wit, only labored gags.”
- ALL of the automated methods fail to beat the original prompt on the SST-2 datasets. This goes to show that you can over-engineer prompts.
- PRewrite outperforms all the other automated methods
Examples
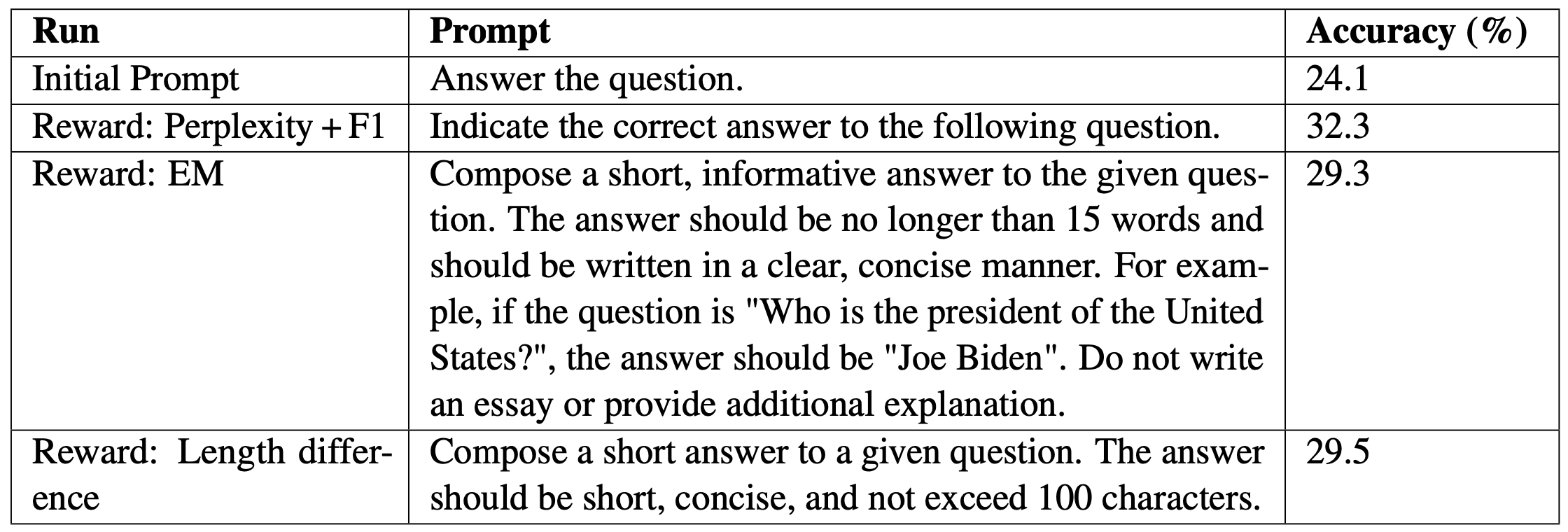
Here are a few examples of how different rewards affect the final rewritten prompt. Again, it isn’t always the longest or most detailed prompt that wins out. In this case, optimizing for Perplexity+F1 leads to the highest accuracy.
There are big changes (~10%) in accuracy depending on the reward mechanism here.

Now the longest and most detailed prompt performs the best, by a few tenths of a point.
What’s most interesting is the difference between the initial prompt and the rewritten prompt with Perplexity + F1 as the reward. They're so similar, yet the performance gap is huge (10%)! Another example showing how subtle changes can make a huge impact in prompt engineering.
Results broken down by Reward type
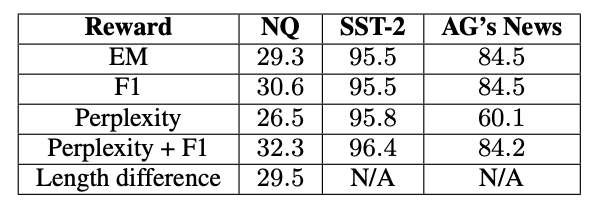
This table comes directly from the paper, but I think it would be more useful to have the performance of the original prompt as well.
- On average, “Perplexity + F1” is the best performing reward for all the datasets
- “Perplexity” performs significantly worse compared to “Perplexity + F1” and is even outperformed by the original prompt in the AG’s News dataset.
- Rewarding for perplexity ensures predictability of responses by the model, but incorporating the F1 score guarantees accuracy too. This addresses both the quality and relevance of content effectively.
Wrapping up
There are a lot of questions around using LLMs to write prompts. Our opinion is that you get the best outputs from combining human effort with LLMs. This new paper provides a new way to think about a framework for automating some of this work, and is definitely worth exploring!
