Google recently dropped a 68-page paper about prompt engineering. It's a nice combination of being very approachable for beginners, covering some of the basics like max token and temperature parameters, while also going into some depth into more complex topics like few-shot Chain of Thought.
The paper is packed with insights and we've added all the example prompts into a single group in PromptHub that you can check out here and start experimenting.
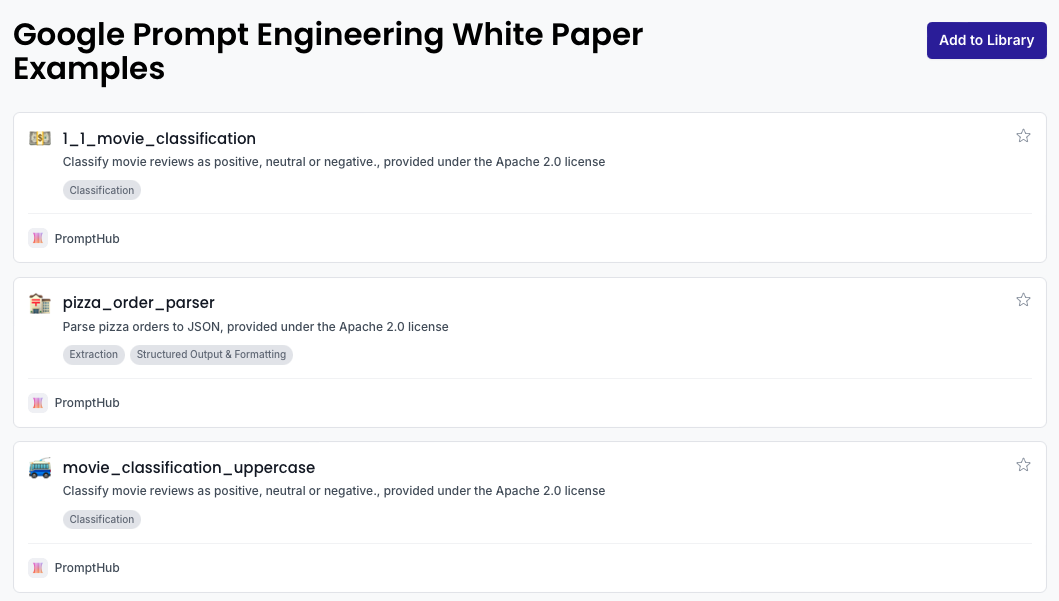
Let’s jump in!
Model parameters
The white paper covers all the core model parameters (for a deeper dive into parameters, check out our guide here: Understanding OpenAI Parameters), but we’ll focus on the three used to dial creativity up or down: Top-P, Top-K, and Temperature.
Top-P
Top-P (nucleus sampling) dynamically selects the smallest set of tokens whose combined probability mass exceeds your P threshold. Lower values (0.5) force the model into a smaller, more predictable token pool. Higher values (0.99) let it draw from essentially all the token options, boosting "randomness" and creative diversity .
Top-K
Top-K sampling truncates to the K highest-probability tokens before sampling. A small K (e.g. 10) means the model has only a handful of choices—great for focused outputs—whereas a larger K (e.g. 40) expands the candidate set and increases variation.
Think of Top-P as probability-mass filtering (you choose a percentage of total probability) and Top-K as fixed-count filtering (you pick the top K tokens by probability).
Temperature
Lower values (<1.0) make the model more deterministic—at 0 it performs greedy decoding, always picking its highest-probability token—while higher values (>1.0) flatten the distribution to boost randomness and diversity in the output .
Example presets
- Default:
P=0.95, K=30, T=0.2
for balanced coherence and creativity. - High creativity:
P=0.99, K=40, T=0.9
expands token diversity. - Deterministic:
P=0.9, K=20, T=0.1
or evenT=0
for single-answer tasks.
Core prompting techniques
The white paper goes over all of the most popular prompt engineering methods and also includes a variety of examples. You can find all the prompt examples mentioned in the paper here, but let’s dive into the methods themselves.
- Zero-Shot Prompting: Provide only a description of the task with no examples. It works best for straightforward tasks where the model already understands the domain (e.g., basic classification or summarization).
- One-Shot Prompting: Supply exactly one example alongside your task description. This single demonstration helps the model understand tone, style, and output format.
- Few-Shot Prompting: Include multiple (typically 3–5) examples to show the desired format, style, or structure. Diverse, high-quality examples are best!
- System Prompting: Give high-level, global instructions about the model’s role or output format (e.g., “Only return valid JSON”). Use it to enforce consistency, structure, or safety guardrails across every response.
- Contextual Prompting: Embed task-specific background information or data (e.g., “Context: You are writing for a retro-games blog”) so the model tailors its output to the right domain or scenario.
- Role Prompting: Assign a persona or job title (e.g., “Act as a travel guide”) to shape tone, vocabulary, and perspective.
- Step-Back Prompting: First ask a broad question to surface relevant background knowledge, then feed its answer into the main task prompt for more robust outputs.
- Chain-of-Thought (CoT): Instruct the model to “think step by step,” generating intermediate reasoning steps.
- Self-Consistency: Run a prompt multiple times under high-temperature sampling and select the most frequent final answer. This majority-vote approach reduces hallucinations and increases reliability.
- Tree of Thoughts (ToT): Explore multiple reasoning branches simultaneously by maintaining a “tree” of intermediate thoughts.
- ReAct (Reason & Act): Combine natural-language reasoning with external tools (search, code execution, etc.) in a thought–action loop, enabling the model to fetch information or run code mid-prompt.
- Automatic Prompt Engineering: Also known as meta prompting. Prompt the model to generate a set of candidate prompts, evaluate them and select the best one.
Best practices
The paper has a ton of best practices scattered throughout. Below are the top ones that stood out, plus here is a link to all the templates so you can start testing them yourself.
- Provide high-quality examples: Few-shot prompting is one of the best ways to teach the model the exact format, style, and scope you want. Including edge cases can boost robustness, but you also run the risk of the model overfitting to examples.
- Start simple: Nothing beats prompts that are concise and clear. If your own instructions are hard to follow, the model will struggle too.
- Be specific about the output: Explicitly state the desired structure, length, and style (e.g., “Return a three-sentence summary in bullet points”).
- Use positive instructions over constraints: Framing “what to do” often beats long lists of “don’ts.” Save hard constraints for safety or strict formats.
- Don’t forget about Max tokens! When you want to constrain output length, use the max tokens parameter.
- Use variables in prompts: Parameterize dynamic values (dates, names, thresholds) with placeholders. This makes your prompts reusable and easier to maintain when context or requirements change.
- Experiment with input formats & writing styles: Try tables, JSON schemas, or bullet lists as part of your prompt. Different formats can potentially lead to better outputs.
- Continually test: Re-test your prompts whenever you switch models or when new model variants are released. As we covered in our GPT-4.1 model guide, prompts that worked well for previous models may need to be tweaked to maintain performance.
- Experiment with output formats: Beyond plain text, ask for JSON, CSV, or markdown. Structured outputs are easier to consume programmatically and reduce post-processing overhead .
- Collaborate with your team: Working with your team makes the prompt engineering process easier. With PromptHub you can share and review prompts in a platform designed for prompts.
- Chain-of-Thought best practices: When using CoT, keep your “Let’s think step by step…” prompts simple. For more reasoning prompts check out this collection in PromptHub here.
- Document prompt iterations: Track versions, configurations, and performance metrics in a centralized platform like PromptHub that will do all the heavy lifting for you.
Putting it all together
Let’s run through a quick example where we want to summarize a product announcement into three bullet points, using all the tips and tricks we’ve learned so far.
1. Simply define the Task
“Generate three concise bullet points summarizing the following announcement.”
2. Choose model parameters
- P = 0.95, K = 30, T = 0.2 for that sweet spot between clarity and creativity .
3. Select prompting technique
- We’ll use Chain-of-Thought (CoT) to guide the model through its reasoning and include a one-shot example to show the model the output format and tone we want.
4. Best practices to keep in mind
- Provide a high-quality example: Show the format and tone we want.
- Be specific about output format: “three bullet points.”
- Use positive instructions: “Generate three bullet points…” rather than “Don’t write paragraphs.”
- Use variables: insert the announcement text as a placeholder so you can reuse this prompt.
5. Sample Prompt
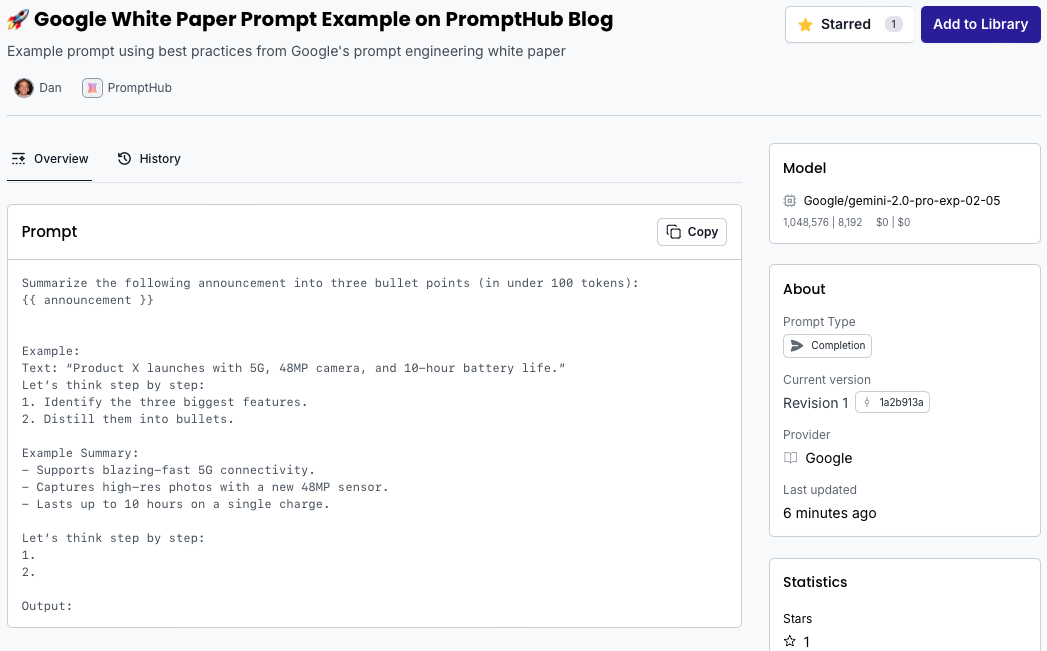
6. Iterate & Test
- Review the bullets for accuracy and tone, test across different models and with different data for the product announcements
- Tweak the model and model parameters
- Log each version and its results automatically in PromptHub
Conclusion
The prompt engineering white paper from Google is an awesome resource for anyone interested in LLMs and prompt engineering. Whether you’re just getting started or have some experience, you'll learn a lot from it!